What is exascale ?
In today’s world, information has become an essential resource. Massive amounts of data are produced every day, from various sources such as social networks, sensors, scientific simulations, and many more. To efficiently process this data and meet the complex challenges of our time, it is crucial to have powerful computing capabilities.
This is where exascale comes in. Exascale is a measure of computing power that represents one trillion (10^18) floating point operations per second, or one million billion calculations per second. This performance is simply astounding and far exceeds that of all existing supercomputers.
Discover the exascale: The computing power of the future
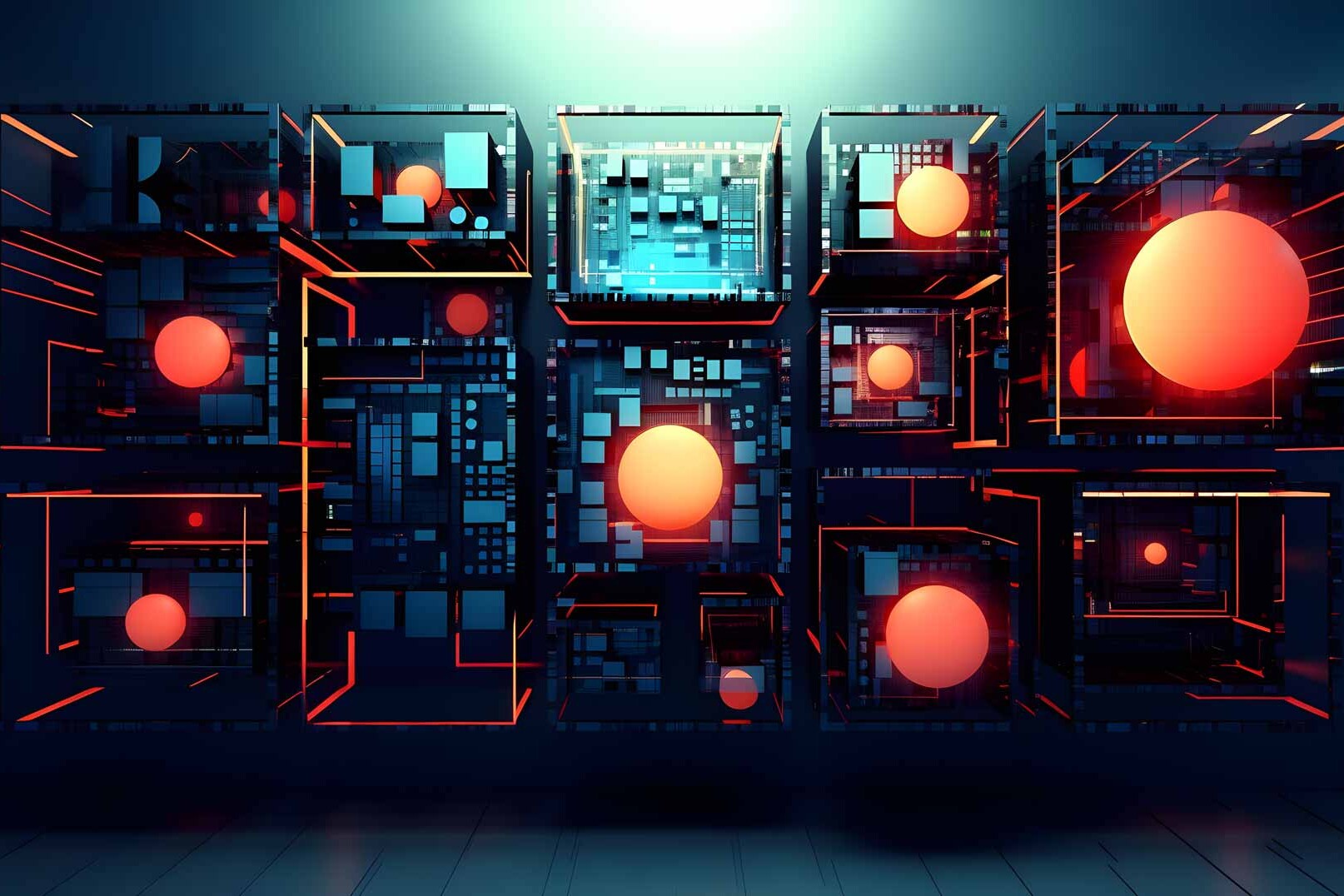
The race to exascale :
Since the first electronic computers, the computing power of machines has grown exponentially thanks to the advancement of technologies. As computational demands grew more complex, researchers and engineers set themselves the goal of achieving exascale. This has given rise to a veritable race for innovation in the field of supercomputers.
Technological challenges :
Achieving exascale is not just about increasing the speed of processors. This requires a multidimensional approach that integrates several research areas. One of the main challenges is to design more energy-efficient processors capable of processing billions of calculations while minimizing power consumption.
In addition, the architecture of supercomputers must be redesigned to fully exploit the performance of processors. Parallel and distributed architectures, as well as the use of specialized processors like graphics accelerators (GPUs), play a key role in achieving exascale.
Exascale applications :
The exascale opens the way to many possibilities in various fields. In science and research, it will enable more accurate and faster simulations, enabling significant advances in fields such as medical research, meteorology, materials physics, astrophysics and many more.
Exascale is also essential for the development of artificial intelligence and machine learning. Deep learning models, which require massive amounts of data and computation, will be able to be trained much faster, enabling faster advancements in these areas.